A Deep Dive into Data-Driven Decision Making
The ongoing digital revolution is transforming the industrialized world in profound ways, with the manufacturing sector being one of the most impacted. Powered by technology, this industry is constantly evolving to meet changing market demands. The advent of advanced tools and technologies such as artificial intelligence, machine learning, IoT, and cloud computing are helping manufacturers optimize production, streamline operations, and make informed business decisions. This wave of transformation is not just a trend; it’s fundamentally shifting the way manufacturers think and operate. In such a rapidly changing environment, the ability to adapt quickly, innovate, and implement operative strategies can make the difference between leading the manufacturing wave or being left far behind. Our new Machine Learning-powered dashboard has been designed with this reality in mind, aiming to support manufacturing businesses in this journey.
Data-Driven Decision Making
In today’s fast-paced manufacturing landscape, making strategic decisions based on hard data rather than mere hunches or our recollection of past experience is critical. Data-driven decision making refers to the act of using quantifiable and verifiable facts to guide business strategies. With the rise in digitization and growing access to consumer and operational data, embracing this approach has become increasingly crucial for manufacturers.
The importance of data-driven decision making in manufacturing unfolds in several key ways. First, it brings precision to business operations – with clear, factual data, manufacturers can predict demand tendencies, adjust inventory levels, manage supply chains, and decrease downtime, thereby increasing productivity and efficiency.
Second, data-driven insights help in identifying and resolving operational inefficiencies. By analyzing production, maintenance, and quality data, manufacturers can uncover potential issues before they escalate into significant problems, ensuring a smooth and seamless production line.
Third, it can enhance our understanding of market trends and consumer behavior. Accurate data analysis can reveal emerging trends and patterns, allowing manufacturers to adapt their operations and products to meet changing consumer demands, and even predict future trends.
Lastly, data-driven decisions can mitigate risks. By tracking and analyzing data related to market changes, uncertainties, or operational challenges, manufacturers can identify risks and develop preventative strategies.
The importance of data-driven decision making within the manufacturing industry cannot be overstated. It’s not just about having the data, but about effectively analyzing and utilizing it to inform strategy, drive productivity, anticipate market trends, and ultimately, remain competitive in an increasingly advanced manufacturing landscape.
The Machine Learning Dashboard
In this example scenario, we’re using 25+ years of historical production data to develop a predictive model of a manufacturing process. See our previous article. for an overview of how this model is constructed. In short, we utilize a portion of the historical data to train a machine learning model, and a separate portion of the data to validate the predictive ability of the model. The model is adjusted until we reach an acceptable level of validation.
The source data includes the components needed to manufacture each part, the steps to produce each part, production data including the time in/out at each production station and the QA pass/fail result, the cost and quantity of components (source materials), part order history, and detailed weekly costs including storage, shipping/handling, and other overhead.
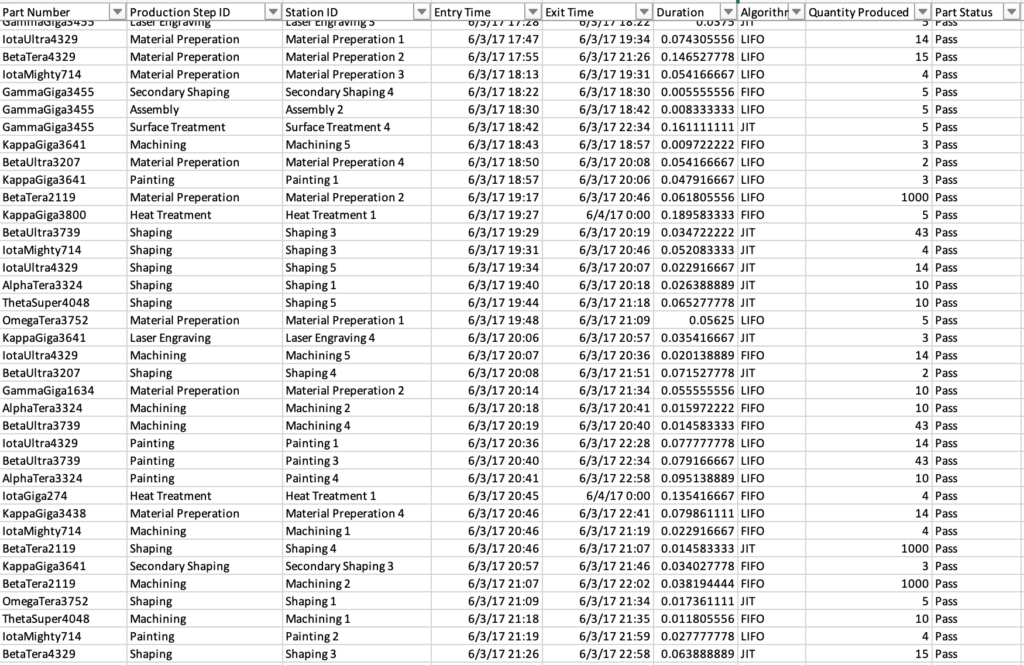
A Snapshot of Production Data
Our primary goals with this analysis are to identify the most and least profitable parts, pinpoint production stages that are the most prone to QA failures, and identify production bottlenecks. Ideally, we’ll also be able to identify which production algorithms (LIFO, FIFO, or JIT) are the most effective and under what conditions, which stations deliver the best and worst QA results, and which parts are the most prone to QA failures. The interesting thing about this analysis is that these are not static data points; these insights are fluid and change over time. Our ML model allows us to roll the clock forward into the future and make predictions about these key metrics to help identify problem areas BEFORE they happen, giving us the information that we need to make key business decisions.
A Specific Example: Where’s the Bottleneck?
From the dashboard image above, it’s not hard to see the problematic production stage (hint: it’s the big green bar), but let’s dig into this a little deeper! What exactly are we looking at here? In short, we have identified a point in the future where the secondary shaping step is slowing down the production process and we’ve got $5.4M of potential profit held up by this friction. Our ML algorithm predicted orders which in turn dictated which parts we needed to manufacture, and based on the model we’re predicting this bottleneck will occur. What you do about this insight depends on the nature of your specific business, but you might increase staffing, run extra shifts, outsource, or even invest in more machinery.
Real Time Monitoring and Retraining
For effective decision making, you’ll want to ensure you’re looking at the most up to date information. In this example, we’re using a static data set but in practice, you’ll want to update your source data as soon as it becomes available; if you have real time monitoring in place to continuously update your data set, even better. You’ll want to automate the inclusion of this new data by constructing an appropriate algorithm to properly clean this source data and optimize it for use by the predictive algorithm.
You’ll also want to periodically retrain your model to continuously validate and improve your prediction capabilities. More data is often better, but you’ll want to carefully validate any new changes to your model to ensure you’re headed in the right direction.
Taking it Further
In our simple example here, we’re utilizing only a fraction of the data available to us. There are undoubtedly other factors that contribute to the efficiency and profitability of the business. For example, how does ongoing staff training affect QA pass rates or machine downtime? Are there specific staff members that might benefit from additional training? Undoubtably more training would lower failure rates and boost operational efficiency, but training costs money and decreases productivity in the short term. If you’re expanding, and adding new and perhaps inexperienced staff, what are the short and long term expectations?
What about sales and marketing spend? How would increased marketing spend influence orders? How would a re-focus on sales affect our close rate? Could we handle a big surge of orders during our busy season? If you’ve got the historical data, these types of what if questions can be within reach with a properly constructed and trained machine learning model.
How might machine maintenance schedules be optimized to reduce unexpected downtime? The answer could be found with a properly constructed ML model.
What unanswered questions do you have about the efficiency and profitability of your manufacturing processes? Comment below and let’s have a conversation!
Subscribe to get the latest in your inbox.
Built with you in mind
Speak to one of our expert consultants about making sense of your data today. During
this free consultation, we'll address your questions, learn more about your business, and
make some immediate recommendations.